Improving Retail Decisions with Customer Analytics
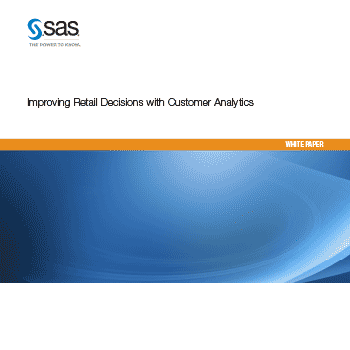
Retailers around the world collect millions of customer data points every day, but most are not making the best use of the vast insights buried within their customer databases. The terabytes of data can become overwhelming.
Retailers quickly drown in customer data and grasp for useful insights. Customer analytics identifies profitable growth opportunities that otherwise go undetected.
Three ways to integrate customer insights into retail decisions are to focus on customer economics, personalized offers and customer-centric merchandising.
Get the downloadBelow is an excerpt of "Improving Retail Decisions with Customer Analytics". To get your free download, and unlimited access to the whole of bizibl.com, simply log in or join free. |
![]() |
Introduction
Retailers around the world collect millions of customer data points every day, but most are not making the best use of the vast insights buried within their customer databases. The terabytes of data can become overwhelming. Retailers quickly drown in customer data and grasp for useful insights. Customer analytics identifies profitable growth opportunities that otherwise go undetected.
Three ways to integrate customer insights into retail decisions are to focus on customer economics, personalized offers and customer-centric merchandising.
Customer Economics
The first step toward integrating customer insights across the enterprise requires customer data exploration and analyses. Understanding your customers’ behavior over time is critical. Two major results from these initial customer data analyses are customer segmentation and customer metrics. These outcomes provide the entire organization with a framework for measuring results and a common customer language across the organization.
Customer Segmentation
Customers can be grouped or segmented using a variety of methods, but fit-forpurpose segmentation enables the incorporation of customer metrics into a variety of business decisions.
Proven customer segmentation approaches include:
- Creation of identifiable segments that merchants understand.
- Focus on segments that reflect corporate strategies.
- Segmentation that is useful for enterprisewide decisions.
Common customer segmentation methods used by retailers include:
- Recency, frequency and monetary (RFM).
- Lifestyle and life stage.
- Price consciousness.
RFM
RFM is a core financial segmentation method deployed by many retailers. Historical purchase behavior found in the customer database is used to group customers by measuring how recently they shopped, how often they shop, how profitable they are and how much they spend.
RFM segmentation quickly identifies the small number of customers who contribute the majority of the sales and profits. For most retailers, the top 20-30 percent of customers account for 50-70 percent of sales and profits.
Customer-centric retailers use this core segmentation method in tandem with typical monthly financial reporting to measure performance across the enterprise.
Lifestyle and life stage
For this segmentation method, you can group customers by behavioral characteristics. Many of these attributes are gleaned from the customer’s shopping history, which is found in your customer database. In addition to shopping behavior for your customers, external demographic and economic data are often incorporated to enrich this view of the customer. These external data elements can include household income, education, household size and presence of children. This segmentation method paints a unique picture of each customer group. Segment names like “Shoppers on a Budget,” “Affluent Professionals,” “Convenience” and “Busy Suburban Moms” are common among lifestyle and life stage segmentations.
Price consciousness
You might want to segment or group customers by sensitivity to price and promotions. The customers’ purchase history within your customer database is analyzed to group customers by answering questions:
- What percentage of items that were purchased was on promotion?
- What percentage of customers’ sales dollars was discounted?
- What percentage of customers’ baskets contained discounted items?
Retailers typically designate these customer groups as subsegments within the RFM or lifestyle and life stage segments. For example, you might strategically tailor promotions to price-sensitive Busy Suburban Moms.
Customer Metrics
After customer segments are in place, the next step toward using customer data across the enterprise is to establish customer metrics. The measurements that answer questions about the retailer’s customers include:
- How many customers shop with us (number of customers or households)?
- How much do they spend each time they shop (sales per week per customer)?
- How much do they buy (items per week per customer)?
- How often do customers shop (visits per week per customer)?
- How profitable are my customers (margin per week per customer)?
- How price sensitive are they (percentage of promoted items purchased/total items purchased)?
These customer metrics are tracked over time and can identify trends such as when your best customers’ purchasing habits change. Armed with customer insights, you can quickly take action to re-engage with those customers.
One way retailers use the customer segmentation and metrics outlined above are in customer-specific promotion offers. Some retailers have millions of customers and thousands of promotional offers per week. They strive to deliver customer-specific offers that are most relevant to each customer.
The sheer number of potential customer-specific offer combinations and the business rules’ complexity exceed the capabilities of most systems. The goal is to offer promotions that are the most relevant based on insights derived from the customer database and are the most profitable for the retailer.
Personalized Offers: Coupon Optimization
Whether online, through the mail or at the register, there is a science to making sure that a coupon is relevant to each customer. Many retailers or their marketing service providers (MSPs) use segmentation, modeling and prioritization to select coupon offers. Only a few use mathematical optimization, but those that do are getting huge returns in both efficiency and profitability.
Interestingly, retailers and MSPs have very different coupon optimization goals. Retailers want to maximize offer redemption (to bring in customers). MSPs want to maximize the number of coupons distributed. Other considerations are contracts with the manufacturers, budget constraints and customer preferences.
Coupon Optimization
Even before we define coupon optimization, it’s important to define the type of optimization that we will discuss in this section.
By optimization we mean using mathematical techniques to find the best possible solution based on certain business rules or constraints. The mathematics goes beyond using sorting techniques and selection criteria, although they have their place and can be effective depending on the circumstances.
In coupon optimization, mathematics is used to maximize results for a goal such as revenue, redemption rate or number of printed coupons. Each of these goals leads to a different outcome. The choice of which to use depends on whether you are a retailer or an MSP. Regardless of the goal, the business rules or constraints that are used in coupon optimization are similar. Next, we will explain the typical scenarios that we find when marketers want to optimize their coupons.
Retailer Coupons
Over the years, several coupon optimization scenarios have emerged, but generally they fall into two categories. The first is direct marketing. You select coupons to be placed in a sheet or booklet format and send them to customers through direct channels – primarily printed mailings.
The second is coupons printed at the point of sale or after customers have paid for their purchases. This second category is similar to how manufacturers and MSPs operate, but we will focus on direct marketing in this section.
In the direct marketing approach, your goal is to get the customer to redeem the coupon and to buy other products while they are in the store. From an optimization standpoint, this means that the objective is to maximize response. Or, if the right data exists, to maximize the revenue or profit from coupon redemption (including total purchase). These optimization values are considered model scores. The optimization software uses the model scores in conjunction with the costs of the offer and other constraints to select the best set of coupons for each customer segment.
Most of the optimization rules or constraints are fairly standard in retail. Retailers have budgets that they have to adhere to and must comply with contracts with manufacturers. These contracts are what can often make an optimization scenario difficult to achieve because there are constraints – for example, a minimum of 2,000 Brand X soda coupons per week. If there are only 3,000 eligible customers, then the constraint becomes restrictive and harder to overcome. The next level of constraints are those within and between brands. For example, a printed coupon sheet can have only four coupons from the soft drinks category, but they cannot be from directly competing brands (see Figure 1).
[Download PDF to see Figure 1]
Note that the last set of constraints above is related to the number of coupons on a sheet. In our example, each sheet or booklet has only 32 spaces, but some coupons take up more than one space, making it even more complex.
A loyalty marketing example
A SAS customer can use SAS® Marketing Optimization to select coupons for a booklet that it distributes periodically to loyalty card members. Each customer can be eligible for as many as 220 offers. The retailer can use business rules and advanced analytics to determine customer eligibility.
A simple rule might identify vegetarian households and exclude any coupons for meat products for those customers. The process of defining eligibility takes place before the data is loaded into SAS Marketing Optimization. By defining eligibility, the offer table is easier to process.
The first task is defining the number of slots available for coupons. Using the previous example of pamphlets with 32 slots for the coupons. If some of the coupons use more than one slot, the optimization analyst has to adjust the scenario parameters (see Figure 1). The analyst does this by adding a column in the communication table (the table that lists all the coupons) with the number of spaces that a coupon will use.
[Download PDF to see Figure 2]
Another constraint for this retailer is category limits. The retailer has a limit of no more than four coupons from any one product group (for example, soft drinks) per customer. These are fairly straightforward constraints, and the analyst creates a constraint for each category.
This situation becomes more complex if the retailer wants to ensure that certain categories are included in the coupon booklet. If the retailer wanted at least one soft drink coupon for every customer, then another constraint would need to be added for that category (i.e., a minimum of one soft drink coupon).
Note that tightening the constraints in this way makes the optimization more difficult (and the scenario runs longer) because the optimization solver has fewer options that meet all the criteria.
The final set of constraints is the minimum and maximum limits from manufacturers. Naturally, manufacturers want to sell more of their products and hopefully gain new customers. The tradeoff is in the manufacturer’s constraints. These constraints are typically at the brand level and often tie to media promotions, so they can change over time.
For example, Brand X might want 10,000 coupons printed in the week leading up to the Super Bowl, but drop to 4,000 the day after the game. Although these kinds of constraints are typically stated in wide ranges, sometimes they are expressed as specific numbers: ”Print exactly 10,000 coupons.” As with the category constraints, these constraints reduce the size of the feasible region, which makes it harder, in general, to find a solution.
All optimization activities must have an objective. In this case, it is to maximize the probability of purchase. This retailer uses a statistical model for optimization to determine this probability.
The simplest measure is the probability of redeeming any coupon, but that does not give the optimization solver as much to work with as would a model for each coupon (or at least for each category). An even more complex objective would be to maximize the value of the customer’s visit based on the probability of redeeming each coupon. This is, of course, a much more difficult task, and most retailers are not able to arrive at these kinds of measures, either due to a lack of skills or the availability of the data.
MSP Coupons
While retailers are tackling the challenges in the above example, some MSPs use SAS to provide coupon optimization as a service at the point of sale (POS).
[Download PDF to see Figure 3]
There are a few important differences between optimizing at the POS and direct marketing. First, we need to define “real time.” So far, no retailers are using “realtime coupon optimization” in the way that we have described. Some might call their process optimization, but the process is not mathematical optimization. It’s not that the technology doesn’t exist, it’s that the focus has not been on the analytics and the data required to pull it off.
For the next example, we will be discussing batch optimization and preselecting the coupons that the customer will receive when they check out, rather than conducting optimization activities in the brief period between the time the cashier totals the purchase and the coupons are printed.
An important difference between POS and direct marketing is the number of coupons involved. Rather than trying to fill a booklet, the retailer only wants a few coupons per customer; often a maximum of five.
The goal is another difference between the retailers and the MSPs. Although the retailers make more revenue when the customer buys more than just the coupon item, that has no effect on the MSP’s revenue. MSPs are often paid by the manufacturers, so the MSP’s revenue depends on the number of printed coupons. The MSP’s goal is to maximize the number of coupons.
Register-printed coupon example
One MSP (a SAS client) had a project for one of their grocery customers in which there were 64 potential coupon offers representing 20 product groups or categories. The grocer had 2 million loyalty card members who were eligible to receive the coupons at check out.
This project is different from the previous retailer example. Rather than printing 32 coupons, the MSP wanted shoppers to get three to five coupons or none at all. The constraint was that each category had a limited number of coupons that could be printed, and each shopper could get only one offer per category.
The objective was to maximize the number of printed coupons while minimizing the number of customers who would not receive coupons. In this case, scoring was at the category level. Because the constraints were less restrictive, the optimization could be completed in a few minutes. This gave the retailer the ability to generate more offers per day, thereby increasing the MSP’s revenue. In addition, the results (number of printed coupons and number of customers receiving coupons) were substantially better than those obtained with a manual prioritization method.
Customer-Centric Merchandizing
Some retail marketing departments use customer analytics to generate offers similar to the ones described above, but only a few retailers are using customer analytics for their merchandising decisions.
For many retailers, customer data is still managed by the marketing department. Customer insights are shared with the merchandising team on an infrequent, fragmented, ad hoc basis via manual reports. This leads to inconsistent use of customer analytics for merchandising decisions.
Two areas in which retailers are beginning to show progress toward integrating customer insights into merchandising decisions are assortments and promotions.
Assortments
Creating localized assortments is the mantra of many retailers. Integrating customer insights into the process of determining which products to offer by location or channel (or both) adds a new dimension to those decisions. Understanding the importance of a product to strategic customer segments is critical information to consider when making drop-or-keep decisions about products.
Retailers are beginning to create loyalty scores that contain customer metrics like loyalty to the retailer, category and brand. Measures of how exclusive a product or brand is to certain customer segments provide guidance in determining whether demand for one product can be transferred to another product. A weighted loyalty score is used in assortment optimization to ensure that lower-selling products that are critical to important customer segments are not discontinued or dropped.
Promotions
Mass promotions advertised in newspaper circulars are still a primary promotional vehicle for many retailers. The process for determining which products to feature and promote on the front page of those circulars is generally cyclical because many decisions are based on the prior year’s promotions.
A few retailers are beginning to incorporate customer insights from their customer database into their ad planning process. They use metrics such as household penetration, revenue per household and units per household to estimate potential sales and profits for the products they are considering for the circular’s front page. These retailers estimate the buying behavior of primary customer segments so overall profitability from the circular is maximized.
Conclusion
We’ve shown in these examples that to optimize insights, retailers can use customer analytics to:
- Incorporate customer insights into key decisions across their corporations.
- Understand customer economics.
- Regularly measure the behavior of each customer segment and understand the related effects on overall business results.
- Use these customer insights when determining strategies.
Some retailers differentiate customer-specific marketing offers and use optimization to ensure that their offers are the most relevant for each customer. And a few of these forward-thinking retailers are beginning to infuse customer insights into their merchandising decisions.
Customer analytics can be a substantial competitive advantage for retailers. We have only scratched the surface of the many ways in which using customer insights can improve customer satisfaction and increase retailer profitability.
Recommended Reading
Davenport, Thomas H. and Jeanne Harris. 2007. Competing on Analytics: The New Science of Winning. Boston, MA: Harvard Business School Publishing Corporation.
About SAS
SAS is the leader in business analytics software and services, and the largest independent vendor in the business intelligence market. Through innovative solutions, SAS helps customers at more than 65,000 sites improve performance and deliver value by making better decisions faster. Since 1976 SAS has been giving customers around the world THE POWER TO KNOW®. For more information on SAS® Business Analytics software and services, visit sas.com.
Want more like this?
Want more like this?
Insight delivered to your inbox
Keep up to date with our free email. Hand picked whitepapers and posts from our blog, as well as exclusive videos and webinar invitations keep our Users one step ahead.
By clicking 'SIGN UP', you agree to our Terms of Use and Privacy Policy
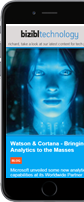
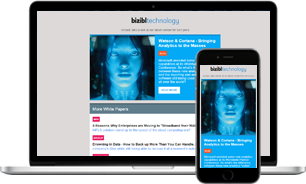
By clicking 'SIGN UP', you agree to our Terms of Use and Privacy Policy